For your organization, data becomes crucial when you use technologies like data analytics, cloud computing, artificial intelligence, and data management systems.
Imagine a world where organizations possess a superpower—an ability to unlock hidden insights, foresee trends, and make decisions with uncanny precision. In this digital era, that superpower is none other than data.
But here’s the twist: data doesn’t wield this power alone. It has a dynamic duo technological marvels that transform raw data into a formidable asset for organizations.
Meet the first contender: Big Data Technologies. These heavyweights, like Apache Hadoop and Apache Spark, can easily handle colossal datasets, sifting through vast amounts of information to uncover hidden gems of insight.
Think of them as the muscle, breaking down barriers and making sure no piece of data goes unnoticed.
Next up is the high-flying acrobat, Data Analytics, and Business Intelligence. Armed with sleek tools and platforms, this contender leaps into action, turning raw data into meaningful patterns and trends.
Data Generation Impact:
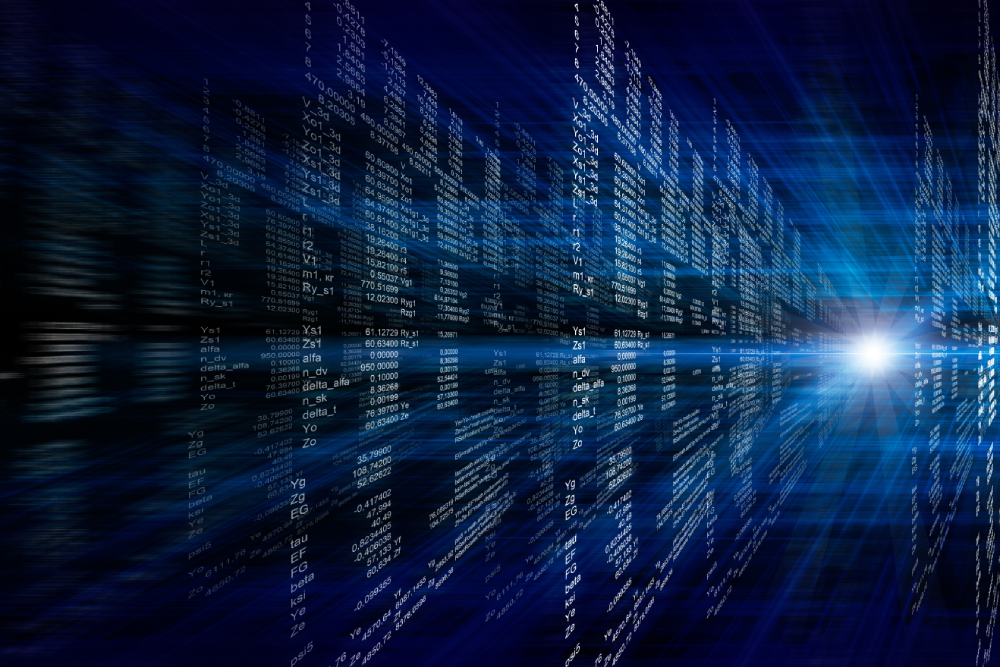
Volume and Velocity: IoT devices generate vast amounts of data in real time, contributing to significant data challenges due to the sheer volume and speed of information.
Diversity: Data from IoT devices is diverse, including sensor readings, location data, and user interactions, providing a holistic view of the environment or system.
Applications:
Smart Homes: IoT devices like smart thermostats, cameras, and appliances enhance home automation, providing convenience and energy efficiency.
Industrial IoT (IIoT): IoT sensors on machinery in manufacturing enable predictive maintenance, reducing downtime and optimizing operations.
Healthcare: Wearable devices and medical sensors track patients’ vital signs, enabling remote monitoring and timely interventions.
Challenges:
Security: With a plethora of connected devices, ensuring the security and privacy of data becomes critical.
Interoperability: Standardization and compatibility issues may arise when integrating diverse IoT devices from different manufacturers.
Advanced Sensors and Data Collection Tools:
Advanced Sensors:
Types: Sensor technologies include optical sensors, environmental sensors, motion sensors, and biometric sensors.
Precision and Accuracy: Advanced sensors provide higher precision and accuracy, crucial for applications like scientific research, healthcare, and autonomous vehicles.
Data Collection Tools:
Drones and UAVs: Equipped with various sensors, drones collect data for mapping, surveillance, and environmental monitoring.
Satellite Imaging: Satellites capture high-resolution imagery for agriculture, urban planning, and disaster monitoring applications.
3D Scanners: Used for precise mapping and modeling, 3D scanners find applications in construction, archaeology, and manufacturing.
Benefits:
Improved Decision-Making: High-quality data from advanced sensors enhances decision-making processes in various industries.
Efficiency: Automation of data collection through advanced tools reduces manual effort and increases efficiency.
Challenges:
Costs: Implementing advanced sensors and tools can be expensive, especially for smaller businesses.
Data Overload: Managing and analyzing the large volumes of data generated by advanced sensors pose challenges in terms of storage and processing capabilities.
Integration of Data Sources for Comprehensive Insights:
Data Integration Strategies:
APIs and Middleware: Application Programming Interfaces (APIs) and middleware facilitate communication and data exchange between different systems.
Data Warehousing: Centralized repositories like data warehouses consolidate information from diverse sources for comprehensive analysis.
Benefits:
A complete view: Integration gives a complete picture of data, helping organizations make smarter decisions.
Real-time Insights: Combining data sources in real-time allows for immediate response to changing conditions or emerging trends.
Use Cases: Customer Relationship Management (CRM): Integrating customer data from various touch points provides a unified view for personalized interactions.
Supply Chain Management: Integration of data from suppliers, manufacturers, and distributors improves visibility and coordination across the supply chain.
Role in Scalable Data Storage:
On-Demand Resources: Cloud platforms offer on-demand access to storage resources, eliminating the need for organizations to invest in and maintain physical infrastructure.
Elasticity: Cloud storage is elastic, allowing organizations to quickly adapt to changing storage requirements without major capital investments.
Benefits:
Cost Savings: Organizations can avoid upfront capital expenses and pay only for the storage they consume.
Challenges:
Security Concerns: Data security and privacy issues must be carefully addressed when entrusting data to third-party cloud providers.
Reliance on Internet Connectivity: Access to cloud storage is dependent on Internet connectivity, which may pose challenges in certain situations.
Database Management Systems for Efficient Organization and Retrieval:
Database Management Systems (DBMS):
Definition: DBMS is software that facilitates database creation, organization, and management.
Types: Relational databases (e.g., MySQL, PostgreSQL), NoSQL databases (e.g., MongoDB, Cassandra), and NewSQL databases.
Efficient Organization:
Data Modeling: DBMS allows for the creation of structured data models, ensuring efficient organization and representation of information.
Indexing: Indexing mechanisms enhance data retrieval speed by creating efficient access paths to database records.
Data Retrieval:
Optimization Techniques: DBMS employs optimization techniques to enhance the performance of complex queries and large datasets.
Benefits:
Data Integrity: DBMS enforces data integrity constraints, ensuring that data stored in the database is accurate and consistent.
Concurrent Access: Supports multiple users accessing and modifying data simultaneously while maintaining data consistency.
Data Quality:
Standards and Policies: Establishing data quality standards and enforcing policies ensures that data is accurate, complete, and consistent.
Data Profiling: Analyzing and profiling data helps identify inconsistencies and anomalies, supporting data quality improvement efforts.
Data Security:
Access Control: Implementing access controls ensures that only authorized users can access sensitive data.
Compliance:
Regulatory Compliance: Data governance practices help organizations comply with data protection regulations and industry standards.
Benefits:
Trust and Reliability: Effective data governance builds trust in the quality and security of organizational data.
Risk Mitigation: By addressing data quality and security concerns, data governance practices mitigate risks associated with inaccurate or compromised data.
Challenges:
Organizational Resistance: Implementing data governance may face resistance from employees who perceive it as additional bureaucracy.
Consistency Across Systems: Ensuring consistent data governance practices across different systems and departments can be challenging in large organizations.
Data Analytics and Business Intelligence
Advanced Analytics Tools for Extracting Meaningful Insights:
Overview:
Advanced Analytics: Involves the use of sophisticated tools and techniques to analyze and interpret complex datasets, going beyond traditional analytics.
Data Visualization: Tools like Tableau, Power BI, and QlikSense help visualize data, making it easier to identify patterns and trends.
Techniques:
Descriptive Analytics: Summarizes historical data to provide insights into what has happened.
Diagnostic Analytics: Examines data to understand why a certain event occurred.
Prescriptive Analytics: Recommends actions to optimize outcomes based on historical and real-time data.
Benefits:
Informed Decision-Making: Advanced analytics tools empower decision-makers with deeper insights, enabling them to make informed and strategic decisions.
Identifying Patterns: Machine learning algorithms within these tools help in identifying hidden patterns and correlations in large datasets.
Challenges:
Data Complexity: Analyzing complex datasets may pose challenges, and ensuring data quality is crucial for meaningful insights.
Machine Learning Algorithms for Predictive Analysis:
Role in Predictive Analysis:
Definition: Machine learning (ML) algorithms analyze historical data to make predictions about future outcomes.
Types: Supervised learning (classification and regression), unsupervised learning (clustering and association), and reinforcement learning.
Applications:
Sales Forecasting: Predictive analytics can forecast sales trends based on historical data and market variables.
Healthcare: Machine learning algorithms help predict disease outcomes, patient responses to treatments, and the likelihood of readmission.
Benefits:
Increased Accuracy: ML algorithms can analyze large datasets and identify complex patterns, leading to more accurate predictions.
Automation: Once trained, ML models can automate the prediction process, saving time and resources.
Challenges:
Data Quality: ML models are highly dependent on the quality of training data, and biased or incomplete data can lead to inaccurate predictions.
Interpretability: Some ML models, especially deep learning models, are often considered “black boxes,” making it challenging to interpret their decision-making processes.
Real-Time Analytics for Agile Decision-Making:
Definition:
Real-Time Analytics: Involves processing and analyzing data as it is generated to provide immediate insights.
Streaming Analytics: Processes data in real-time streams, enabling quick response to changing conditions.
Applications:
Financial Trading: Real-time analytics is crucial for making split-second trading decisions in financial markets.
Supply Chain Management: Monitors and optimizes logistics, inventory, and production processes in real time.
Benefits:
Agile Decision-Making: Real-time analytics allows organizations to respond promptly to emerging trends, issues, or opportunities.
Operational Efficiency: Continuous monitoring of data streams improves operational efficiency by identifying and addressing issues in real-time.
Challenges:
Data Latency: Ensuring low-latency processing is essential to provide truly real-time insights, and delays may impact decision-making.
Integration of Artificial Intelligence (AI)
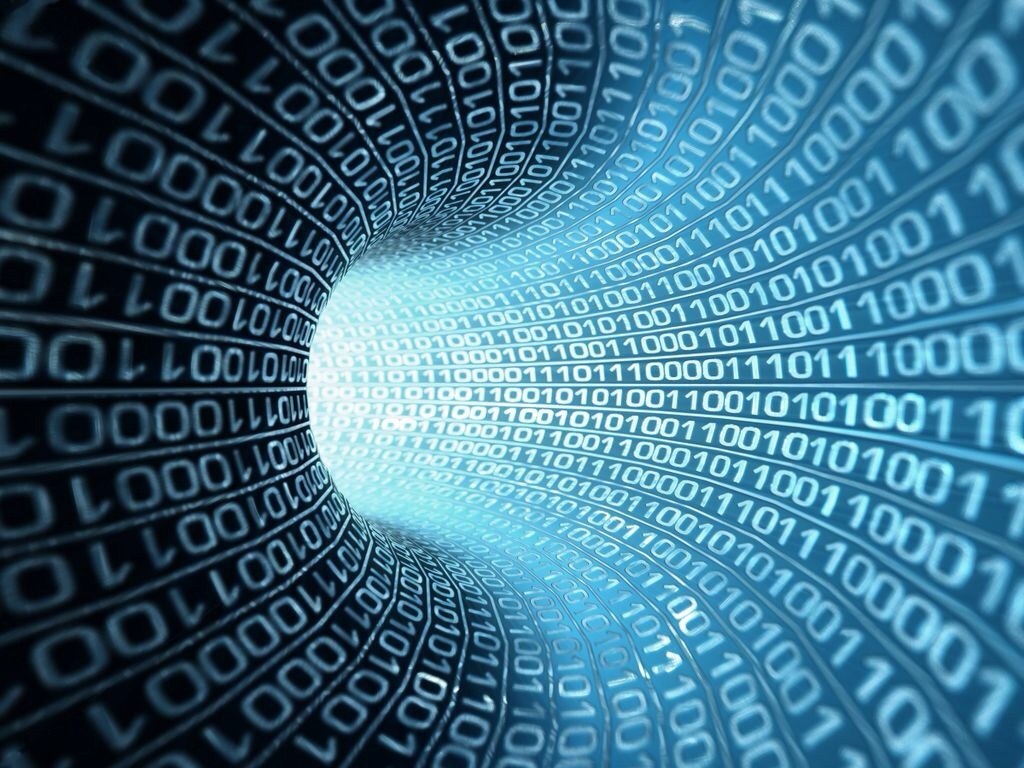
AI-driven Automation for Data Processing and Analysis:
Automation in Data Processing:
Routine Tasks: AI automates repetitive tasks in data processing, such as data cleaning, transformation, and normalization.
Data Preparation: AI algorithms can automatically preprocess and organize data, saving time and reducing human error.
Automated Analysis:
Pattern Recognition: AI systems can more efficiently identify patterns, trends, and anomalies in large datasets than traditional methods.
Predictive Analytics: Machine learning algorithms automate the process of building models for predictive analysis.
Benefits:
Consistency: AI ensures consistency in data processing and analysis, minimizing the risk of human errors.
Challenges:
Data Quality: Automated processes are only as good as the quality of the input data, and ensuring data quality remains a challenge.
Ethical Considerations: Ethical concerns may arise when decisions based on automated analysis impact individuals or groups.
Natural Language Processing (NLP) for Extracting Insights from Unstructured Data:
Definition of NLP:
Natural Language Processing: NLP, a part of AI, concentrates on how computers and human languages interact. It empowers machines to comprehend, interpret, and create text that resembles human language.
Text Analysis:
Sentiment Analysis: NLP can analyze text data to determine the sentiment expressed, which is valuable for understanding customer feedback and opinions.
Entity Recognition: NLP identifies and classifies entities (e.g., names, organizations) within unstructured text.
Benefits:
Insights from Unstructured Data: NLP enables the extraction of valuable insights from unstructured data sources, such as social media, emails, and articles.
Improved Search and Information Retrieval: NLP enhances search engines by understanding user queries and providing more relevant results.
Challenges:
Ambiguity and Context: Understanding the context and ambiguity in natural language can be challenging for NLP systems.
Bias in Language: NLP models may reflect and perpetuate biases present in training data, raising ethical concerns.
AI’s Role in Personalization and Customization of Organizational Strategies:
Personalization in Marketing:
Recommendation Systems: AI analyzes user behavior to provide personalized recommendations, enhancing customer engagement.
Targeted Campaigns: AI helps tailor marketing campaigns based on individual preferences and behaviors.
Customization of User Experiences:
User Interfaces: AI-driven interfaces adapt to user preferences, providing a personalized and intuitive experience.
Product Customization: AI assists in customizing products or services based on customer needs and feedback.
Organizational Strategies:
Dynamic Pricing: AI enables dynamic pricing strategies based on real-time market conditions and customer demand.
Resource Allocation: AI optimizes resource allocation by analyzing data to identify areas for improvement and efficiency.
Benefits:
Customer Satisfaction: Personalization enhances customer satisfaction by delivering tailored experiences and offerings.
Competitive Advantage: Organizations leveraging AI for personalization gain a competitive edge by meeting individual customer needs more effectively.
Challenges:
Balancing Customization and Standardization: Striking the right balance between personalized experiences and maintaining standardization can be challenging for organizations.
Data Security Technologies
Encryption and Blockchain for Securing Sensitive Data:
Encryption Overview:
Types: Symmetric encryption (same key for both encryption and decryption) and asymmetric encryption (public and private key pair).
Role in Data Security:
Data in Transit: Encrypting data during transmission (e.g., HTTPS for web communication) protects it from interception.
Data at Rest: Encrypting stored data on devices or servers safeguards it from unauthorized access.
Blockchain for Data Security:
Decentralized Ledger: Blockchain provides a decentralized and tamper-resistant ledger, ensuring data integrity.
Smart Contracts: Self-executing smart contracts on blockchain platforms enhance the security and automation of data transactions.
Benefits:
Integrity: Blockchain’s immutability ensures that data remains unchanged and trustworthy.
Challenges:
Key Management: Managing encryption keys is crucial for effective data protection.
Scalability: Implementing blockchain can face challenges in terms of scalability, especially for large-scale applications.
Identity and Access Management to Control Data Access:
Identity and Access Management (IAM) Overview:
Definition: IAM involves managing and controlling user access to systems and data based on their identities and roles.
Components: User provisioning, authentication, authorization, and access auditing.
Role in Data Security:
Authentication: Verifying the identity of users through factors like passwords, biometrics, or multi-factor authentication.
Authorization: Defining and managing access rights based on user roles and responsibilities.
Single Sign-On (SSO):
Reduced Credential Management Risks: Users manage fewer credentials, reducing the likelihood of weak passwords or password reuse.
Benefits:
Reduced Insider Threats: IAM helps mitigate insider threats by ensuring users have only the necessary access rights.
Compliance: IAM frameworks assist organizations in meeting regulatory compliance requirements related to data access.
Challenges:
User Lifecycle Management: Effectively managing user access throughout their lifecycle, including onboarding, role changes, and offboarding.
User Experience vs. Security: Balancing the need for strong security by providing a seamless user experience can be challenging.
Cybersecurity Measures to Protect Against Data Breaches:
Firewalls and Intrusion Detection Systems:
Firewalls: Regulate incoming and outgoing network traffic according to established security rules.
Intrusion Detection Systems (IDS): Monitor network or system activities for signs of malicious behavior or security policy violations.
Endpoint Security:
Antivirus Software: Identifies and eliminates harmful software from endpoints, stopping the spread of malware.
Endpoint Detection and Response (EDR): Monitors endpoint activities and responds to security incidents in real-time.
Incident Response and Threat Intelligence:
Incident Response Plans: Organizations develop and implement plans to respond effectively to security incidents.
Threat Intelligence: Utilizing information about current and potential threats to enhance proactive cybersecurity measures.
Employee Training and Awareness:
Phishing Awareness: Educate employees about phishing attacks and social engineering tactics to prevent unauthorized access
Benefits:
Early Detection: Cybersecurity measures help detect and respond to security threats at an early stage, minimizing potential damage.
Comprehensive Defense: A combination of cybersecurity measures provides a layered defense, making it more challenging for attackers to breach security.
Challenges:
Evolving Threat Landscape: Adapting cybersecurity measures to keep up with the constantly evolving tactics of cyber attackers.
Resource Constraints: Organizations may face challenges in allocating sufficient resources for robust cybersecurity infrastructure and practices.
The Role of Data in Digital Transformation
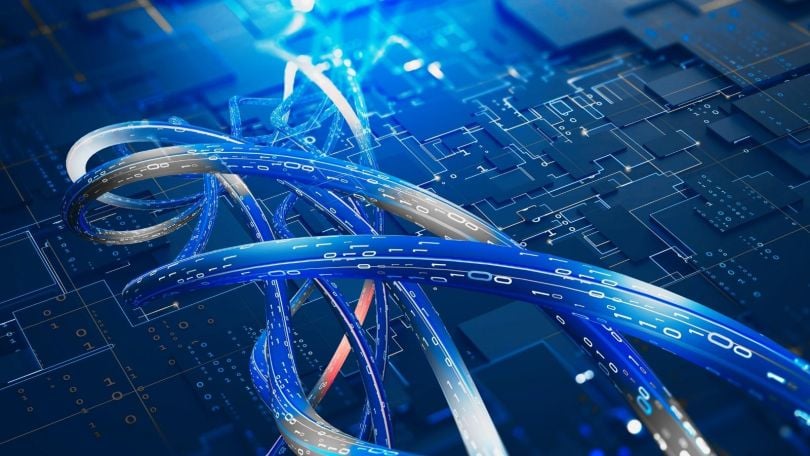
How Data Becomes a Catalyst for Organizational Innovation:
Data-Driven Insights:
Understanding Customer Behavior: Analyzing customer data helps organizations gain insights into preferences, buying patterns, and user experiences.
Informed Product Development:
User Feedback and Iteration: Data from user interactions and feedback loops inform product development, allowing for iterative improvements based on actual usage patterns.
Predictive Analytics: Anticipating customer needs and preferences through predictive analytics guides innovation in product and service offerings.
Efficiency Improvements:
Process Optimization: Data-driven insights highlight process inefficiencies, leading to streamlined operations and increased organizational agility.
Automation Opportunities: Identifying repetitive tasks through data analysis paves the way for automation, freeing up resources for more strategic endeavors.
Agile Decision-Making:
Real-time Analytics: Access to real-time data enables agile decision-making, allowing organizations to respond promptly to opportunities and challenges.
Experimentation Culture: Data empowers organizations to foster a culture of experimentation, where hypotheses are tested and innovation is encouraged.
Case Studies of Organizations Leveraging Data for Transformation:
Amazon:
Recommendation Algorithms: Amazon leverages data to power recommendation algorithms, driving personalized shopping experiences for users.
Supply Chain Optimization: Data analytics is used for supply chain management, ensuring efficient inventory levels and timely deliveries.
Netflix:
Content Personalization: Netflix uses data to analyze user viewing habits and preferences, offering personalized content recommendations.
Content Production: Data informs decisions on content creation, helping Netflix invest in original programming based on audience demand.
Tesla:
Autonomous Driving: Tesla collects vehicle data to improve autonomous driving capabilities, using machine learning to enhance safety and performance.
Over-the-Air Updates: Data-driven insights enable Tesla to deploy over-the-air updates, improving vehicle functionality and addressing issues remotely.
Uber:
Dynamic Pricing: Uber employs data analytics for dynamic pricing, adjusting ride costs based on demand, supply, and other variables.
Route Optimization: Data-driven algorithms optimize routes, reducing travel time and enhancing the efficiency of the Uber platform.
The Impact of Data-Driven Decision-Making on Overall Business Strategy:
Strategic Alignment:
Data-Driven Culture: Organizations with a data-driven culture align their strategies with insights derived from data analysis.
Strategic Planning: Data informs strategic planning processes, ensuring alignment with market trends and customer expectations.
Operational Excellence:
Efficient Resource Allocation: Data-driven decision-making optimizes resource allocation, directing investments and efforts toward areas with the highest impact.
Process Improvement: Data identifies operational bottlenecks, enabling organizations to implement process improvements for enhanced efficiency.
Customer-Centric Approaches:
Personalized Marketing: Data-driven insights allow for personalized marketing strategies, tailoring messages and offerings to individual customer segments.
Enhanced Customer Experience: Understanding customer behavior through data informs initiatives aimed at improving the overall customer experience.
Risk Management:
Predictive Analytics for Risk Assessment: Data-driven models predict potential risks, allowing organizations to mitigate and manage risks proactively.
Compliance and Security: Data-driven strategies address compliance requirements and enhance security measures to safeguard sensitive information.
Innovation and Adaptability:
Continuous Improvement: Data-driven decision-making fosters a culture of continuous improvement, encouraging organizations to adapt to changing market dynamics.
Innovation Pipelines: Data guides the innovation pipeline, ensuring that new ideas are grounded in actionable insights and market demand.
In summary, the role of data in digital transformation is multifaceted, impacting organizational innovation, driving strategic decisions, and facilitating agility in the face of dynamic business environments.
Successful organizations leverage data as a valuable asset and a core driver of transformative initiatives.
FAQs
What technologies make data a critical asset for organizations?
Answer: Technologies such as cloud computing, advanced analytics tools, and database management systems play a crucial role in making data a valuable organizational asset.
How does cloud computing contribute to the importance of data for organizations?
Answer: Cloud computing provides scalable and flexible storage, allowing organizations to manage and access their data efficiently, making it a critical asset.
What role do advanced analytics tools play in leveraging data for organizations?
Answer: Advanced analytics tools help organizations extract meaningful insights from data, supporting informed decision-making and driving innovation.
Why are database management systems essential for organizations to consider data valuable?
Answer: Database management systems organize and manage data efficiently, ensuring its integrity and accessibility, making it a critical asset for organizations.
How does the Internet of Things (IoT) contribute to the significance of data in organizations?
Answer: IoT devices generate vast amounts of real-time data, providing organizations with diverse and valuable insights and making data a critical asset.
In what ways do machine learning algorithms enhance the value of data for organizations?
Answer: Machine learning algorithms analyze data to predict outcomes, personalize experiences, and automate decision-making, adding immense value to data for organizations.
What impact do encryption and blockchain technologies have on securing sensitive data?
Answer: Encryption and blockchain technologies ensure the security and integrity of sensitive data, making them essential for safeguarding this critical organizational asset.
How does identity and access management contribute to controlling data access and protection?
Answer: Identity and access management (IAM) ensures only authorized individuals have access to data, playing a crucial role in protecting and managing this organizational asset.
What is the significance of real-time analytics in making data a valuable asset for organizations?
Answer: Real-time analytics enables organizations to respond promptly to changing conditions, leveraging data as a dynamic and actionable asset.
Why is data governance important for organizations aiming to treat data as a critical asset?
Answer: Data governance establishes policies and practices for data management, ensuring its quality, security, and strategic use as a valuable organizational asset.
How do organizations ensure ethical use of data, and why is it important to consider data as a critical asset?
Answer: Organizations ensure ethical use of data through clear policies, ethical guidelines, and employee training, as it is vital for maintaining trust and integrity with this valuable asset.
Conclusion
Remember a few things when diving into the world of data. First, make sure you’re playing by the rules – keep an eye on privacy and follow the regulations.
Break down those walls between different teams and systems so everyone can work together smoothly.
And most importantly, always use data fairly and honestly – think about the impact on people. By doing these things, you’ll handle data wisely and open the door to exciting possibilities for your organization.